Eventful
Event modelling for social good
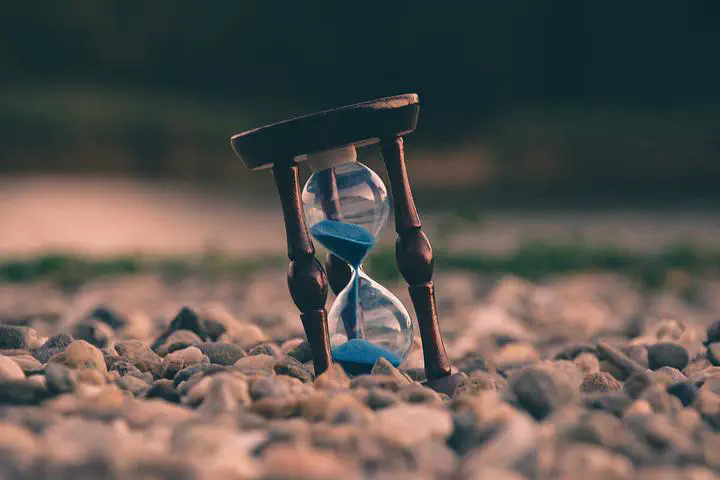
An ‘event’ can describe any state with a timestamp. Some events are directly observable (death, crime reports, flooding) while others represent latent changes in an underlying process (flares in disease, social unrest, a cyber-attack). Predicting events—when, whether and where they occur—is an increasingly important task in science, industry and public policy.
In the world of complex crises (i.e. a combination of extreme climate hazards, new pandemics and political polarisation leading to increased warfare and civil unrest), understanding and modelling these crises is essential for mitigating the impacts on all aspects of life, including health, education and risk from violence. Informing data-driven evidence based policy making and interventions will require new approaches to multilayer modelling, taking into account economic, political, geographical, climate, and myriad of other event types. Rarely are these events recorded to the same level of geographical or temporal granularity, adding an additional level of complexity.
This project aims to extend, combine and improve state-of-the-art methods used to model events to increase their performance, explainability, trustworthiness and applicability to richer data sets. We will tackle different and new data cleaning strategies, work on improving the way data is binned and how surveys are performed, and in particular work to model complex spatio-temporal patterns, for instance predicting future rates or counts of crimes reported in different locations. We also aim to improve explainability of models for complex event modelling, and open source our solutions so that they can be used in academia, policy making and beyond.
This project is a perfect fit for you if you have some experience in spatio-temporal modelling, statistical modelling, deep learning, and/or working with different data types.