Stein's method for Statistics and Machine Learning
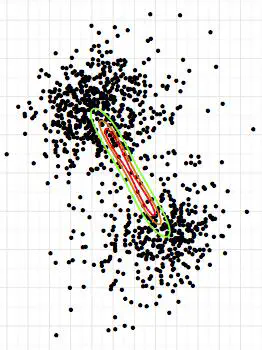
Stein’s method is a probabilistic approach to controlling the distance between probability measures in terms of a differential and difference operators. While this method was first developed with the aim of obtaining quantitative central limit theorems, it has recently been adopted by the computational statistics and machine learning community for various practical tasks including goodness-of-fit testing, generative modeling, global non-convex optimisation, variational inference, de novo sampling, constructing powerful control variates for Monte Carlo variance reduction, and measuring the quality of Markov chain Monte Carlo algorithms.