curATime
cluster for atherothrombosis and individualized medicine
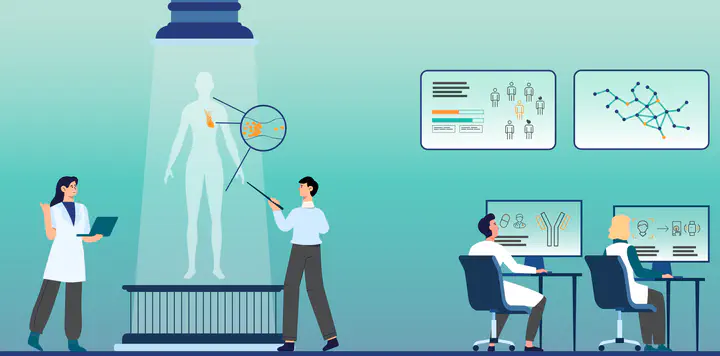
curATime is a collaborative, multi-centre effort applying AI methods to high-dimensional biomedical data to promote an understanding of biological processes associated with cardiovascular illness.
This exciting project involves highly granular data collected as part of the Gutenberg Health Study — a prospective cohort study of a representative sample (
Our primary goal is to understand processes behind cardiac events, including atherosclerosis and atherothrombosis. This depends upon a comprehensive characterization of clinical samples, to decipher complex pathophysiological mechanisms in different disease settings. Hence the task—at the confluence of modern biomedical research and artificial intelligence—will exploit multi-dimensional datasets of protein, lipid and metabolite levels, together with bioinformatics workflows, machine learning and multi-omics data integration.
Contributions to the project involve the following:
- Identifying opportunities for applications of AI to improve multi-omics research
- Development of reusable machine learning pipelines for bioinformatics research
- Extending statistical methodology to new areas and regimes, especially evaluating existing methods for classification to time-to-event prediction tasks
- Collaboration with domain experts, including biostatisticians and clinicians
- Dealing with limitations of sparse and sensitive data, through appropriate use of ‘small data’ approaches, for example data augmentation, synthetic data generation, transfer learning, Bayesian models or use of knowledge graphs.
This project is a perfect fit for researchers with experience or interest in working with high-dimensional, multi-modal data structures, deep learning, probabilistic reasoning and/or biomedical knowledge graphs.